Connect with our experts for counseling on your next step to succeed in your career. Start your 30-day free trial to access over 900 courses.
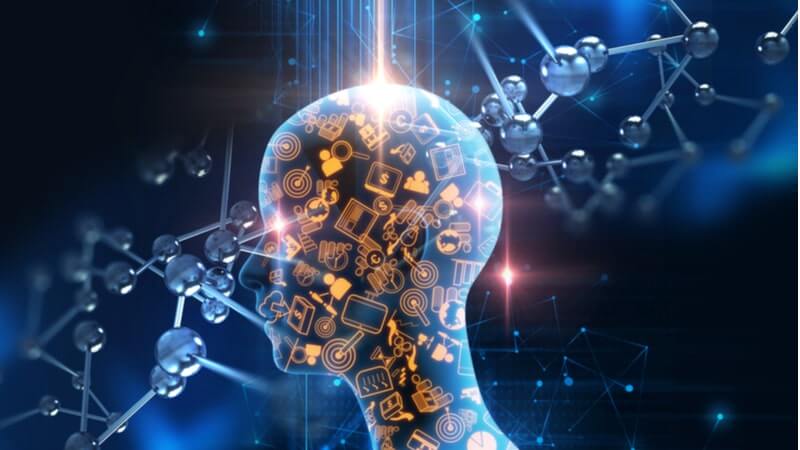
How to Get into a Top AI & ML Bootcamp in 2021
Today’s world is quickly growing with the advancements of technology. The significance of both machine learning and artificial intelligence has been evolving through the years. We witness an increasing number of organizations are making use of these technologies. They are using these tools to enhance their services and products, assess their business frameworks, and also upgrade their process to make decisions.
From automatic cars to the android systems in many smartphones, applications, and electrical devices, ML and AI have an extensive range of influence nowadays. We see AI & ML are transforming our lives by enabling machinery to support us in our jobs and daily tasks. The industry is progressing fast, and the requirement for ML and AI engineers is also evolving. Connect with our experts to learn more about our Data Science and Analytics Bootcamp.
What Are Artificial Intelligence Degrees?
The degree of Artificial Intelligence allows professionals to be able to generate intellectual systems and mechanisms. These systems perform tasks that would require human intelligence - such as playing games or else understand natural linguistic. The topmost renowned samples of Artificial-Intelligence consist of chess-playing computers and self-driving cars. They rely on deep learning and natural language processing to analyze large volumes of data, identifying patterns, and making estimates and conclusions reliant on relevant info.
What Are Machine Learning Degrees?
Machine Learning seems to be a more specialized sub-discipline of AI. The degree of Machine Learning teaches candidates ways to utilize algorithms and models of stats to generate computer networks that would be learned for them. These kinds of computer networks utilize data, as well as self-generated reactions that are sample and fault to keep performing the tasks, and there would not be any instructions from systems analysts.
One of the core examples of a system of Machine Learning is software that recognizes a picture used by organizations such as Apple or Google. The network examines the inner elements of pictures and - then assembles them in other categories such as color, subject, and location.
AI Engineer vs. ML Engineer
AI and ML engineers work under the shared roof of AI. However, their accountabilities and job roles vary somewhat and are reliant on strategies and tools in usage, and results. Artificial intelligence emphasizes the probability to ensure over accurateness. On the other side, the engineers of AI make use of data for decision-making. However, the engineers of ML learn the newest elements from data.
AI engineers use C ++, Java, and different tools for software development. Though, the engineers of ML are needed to know the algorithms and data tools, like TensorFlow and H2O. Both job roles attain a similar output and make use of different strategies. Many top organizations are employing experts proficient in both artificial intelligence and machine learning.
Getting into an AI and ML Bootcamp - 2021
The major dissimilarity between ML and AI is that AI consists of the entire systems, methodologies, and machines that perform smart actions. The focus of ML is only on the methods of AI and apps that learn and alter their patterns of decisions reliant on the data. We suggest beginners follow these steps to get into a bootcamp:
Break All Barriers to Approaches
You can practice and implement machine learning and artificial intelligence with actual life examples in the real-world. All of this is started with just an idea and using machine learning. You then bring that idea into action. You should keep extending your mind to develop more skills. In case you get this exciting, tell yourself these things:
- What keeps you separate from your goals in machine learning and artificial intelligence?
- How do you think regarding the concerns with machine learning and artificial intelligence?
- How would a trainer support you in breaking down your problems?
Find an optimistic meeting group for machine learning and artificial intelligence and get connected with them.
Have a Process
Use this methodology to keep working on the challenges with machine learning and artificial intelligence.
- Find out the problem and hold it.
- The methodology will appear similar to this.
- Evaluate the alterations to the set of rules.
- Turn out the set of data ready.
- Develop the results of data.
- Signify the assumptions to the shareholders.
Select a Tool for Programming
- Select a toolset for a specific stage and use it according to your way.
- Workbench is utilized for learners.
- Intermediate: Python Ecosystem.
Practice Your Abilities
- Select the set of data to keep working on, and utilize the methodology for practicing on it.
- Practice machine learning with in-memory archives. You might also consider actual sets of data of machine learning, and then practice with them. Their goal is to take along these little sets of data into action. In such a manner, you will be switching to larger, more difficult sets of data.
Develop Your Performance into a Good Portfolio
- As soon as you have to be acquainted with your abilities, demonstrate to the organizations that you comprehend what methods of machine learning and artificial intelligence you will develop.
Artificial and Machine Learning - Common Skill Set
Programming Languages
A better understanding of software design languages, such as C++, Java, R, and Python is essential. They are easily learned, and their apps offer more scope compared to a different language. Python is the undoubted lingua franca of ML.
Linear Algebra, Calculus, Statistics
It’s suggested to have detailed knowledge regarding concepts of matrix multiplication vectors and matrices. Furthermore, understanding integrals and derivatives and their implementation are necessary to even comprehend the simplest concepts, such as gradient descent.
In the meanwhile, the concepts of stats, such as Gaussian distributions, standard deviations, and mean should be learned. However, the theory of probability for the algorithms, such as the Hidden Markov Model, Gaussian mixture models, and naive Bayes is essential to acknowledge.
Signal Processing Techniques
You also must have the expertise to understand signal processing and the capability to resolve numerous issues. Making use of signal processing methods is vital for feature-extraction. Though, the feature extraction is a significant phase of ML. Afterward, we possess time-frequency analysis along with progressive signal processing algorithms. A deep theoretical and applied knowledge will assist you to resolve critical scenarios.
Applied Math and Algorithms
Knowledge of the detailed fundamentals and proficiency in the theory of algorithm is a must-have. This set of skills allow understanding subjects such as summations, partial differential equations, quadratic programming, LaGrange, convex optimization, and gradient descent.
Network Architectures
ML is utilized for critical tasks which are away from the capability of humans to code. Thus, networks are being understandable and verified by the most detailed way to counter several concerns, such as classification of the image, recognition of speech, and translation, which are acting as key roles in the domain of AI.
Interaction
Interaction is the core aspect in any domain of work, even in artificial intelligence or machine learning engineering. Explaining the concepts of artificial intelligence or machine learning to the layman is only imaginable once they communicate smoothly and clearly. The ML and AI engineers do not work alone. Projects involve working together with engineers as well as non-technical members of teams, such as sales or marketing departments.
Fast Prototyping
It’s not easy to work with a perfect idea spending less time on it. Particularly in ML, selecting the relevant model and working on projects, such as A/B testing, are keys to a successful project. Rapid prototyping assists in forming other kinds of methods to securely generate a measurable model.
Reinforcement Learning
It is observed recently that reinforcement learning was the actual cause behind enhancing Deep Learning and AI to a wider range. Reinforcement learning performs as assistance to cover the way in an automation field, such as self-driving cars and artificial intelligence domains. There are actual world circumstances that necessitate implementing ML strategies to the systems, and all this happens once the understanding of physics is implemented.
Computer Vision
Computer vision (CV) and ML are considered the two main computer science branches. They are individually working and controlling critical systems and networks that are completely relying on computer vision and machine learning algorithms.
Wrap-Up
Every single thing is initiated to some extent, like an idea in a person’s mind. It is easier to overlook that when types of mechanism and computer networks can perform the best things, and it is the human mind which develops, maintains and generates them. That is the reason why industries must be playing an active role to support their current workers via re-skilling or by offering programs, such as AI and ML training, or by encouraging them to get into a bootcamp. In that way, individuals are taking an active approach towards their lifetime knowledge. It seems to be a crucial and critical task of our time.
Since machine learning and artificial intelligence are both essential, we should use them for the betterment of the world Moreover, we must have an insight and awareness of the program and utilize these newest technologies properly so that it benefits our planet and people, and not only for the monetary interests of businesses.