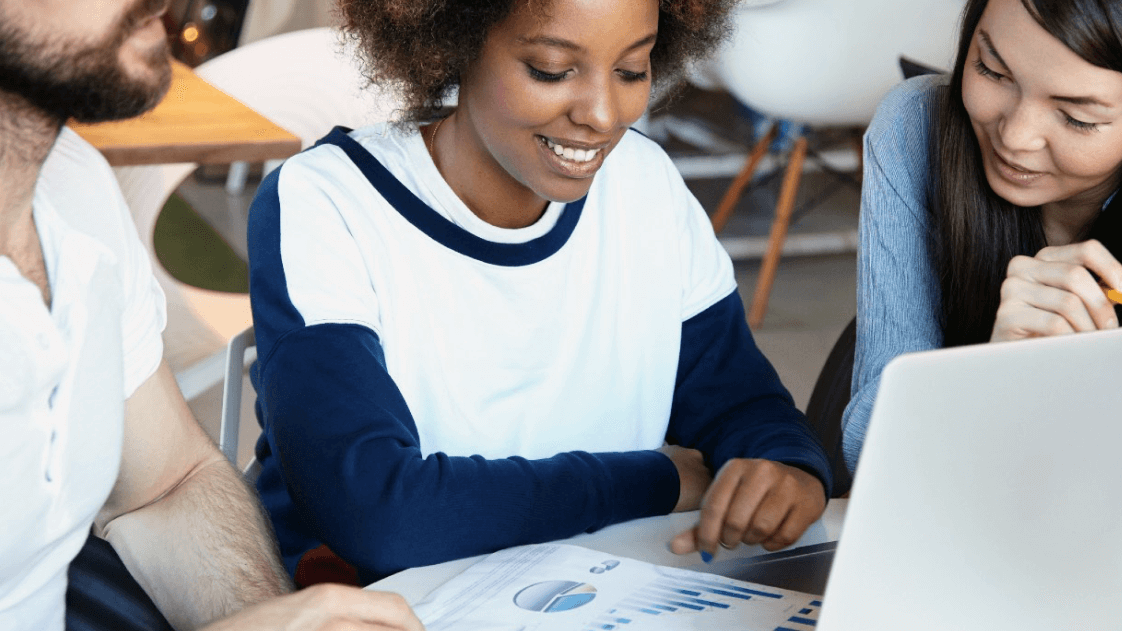
How to plan successful data science projects and how to evaluate success?
Data is almost everywhere and it comprises a variety of systems on a day to day basis. Politicians use that data to win the elections, marketers and businessmen use this data to increase their overall sales, and thus along with it the profitability of their franchises and athletes use this data to win at leagues and perform their absolute best. We as a matter of fact are fully covered with data, small data, big data, raw data, and processed data, this is what our digital world is made of.
Despite such large aptitude for digital data the data science projects that are created and developed don't perform that well and often miss on their mark. Therefore, when it comes to data science project planning you must be looking out for plenty of things and elements to get by. The data science success evaluation is something you must do prior to designing the project and the following tips might help you to do just that;
Estimating ROI before starting out
When it comes to starting the data science project the first mistake that many teams make is to start out on their plan without first estimating its overall impact and comparing it with other available options. The best way you can be done with it is like dealing with any other investment-oriented decision. You start by considering all possible and available options that are actionable too, evaluating the costs and the benefits that can come along with it. When you have processed all that information only then you can come across the likelihood of choosing the most attractive option out of the lot.
Another key factor that you must absolutely be looking forward to is the potential for impact. Such as choosing between the customer churning or better prospect targeting then you must think for the potential that lies between the two and who has more to offer. Think about which one between the two would incur fewer costs and which one is likely to deliver more percentage in return as ROI to the total investment done? When you have derived the pros and cons only then you can go for the best option available to you.
Start small and then add to it
With the data science projects that you might have designed the temptation to overinvest might take you in the long run but it is advised that you refrain from doing it. A thoughtful consideration in this aspect can be when having to buy the equipment for your data science project, vendors might want you to go with overpriced and expensive gadgets that can often backfire with no prospects of an ROI returning your way whatsoever. So, the most obvious thing to do here would be to start small, design your projects in such a way that the data science success metrics remain tempting enough through and through. This you the data science project management can also become way easier while having to deal with fewer and fewer things to cater to.
So, if you were thinking that starting small means the project is unimportant or you wouldn't be able to hit any mark with it then clearly you are in the wrong. Such small projects are able to generate a compelling amount of ROI and produce effective insight that can be used for future predictions. The best advice that you can follow or is recommended is that you must initiate a data science project that is expected to deliver at least ten times more ROI and only takes almost three to four months to complete.
Implementation of the plan
Only conceptualizing a project is not going to turn your project into an absolute success, what you also require within the recipe is the perfect execution of this plan. Talking about the very foundation of data science; it processes and generates data as well as working on putting important decisions out there. So, in its essence, it is a two-step process in which the insights are first generated and later these are acted upon.
Many companies or organizations progressing with this implementation of their plan will totally ignore these insights and won’t act upon them. No matter how important or visually impressive that insight looks it still needs to be acted upon. So, from the get-go you must have in mind the various concepts that you can act upon, what are the insights that can be considered and what tools can eventually help you to get on with this current plan? You must have all of this figured out in advance or otherwise the data science project that you are going to work on is destined to be doomed.
Stakeholder involvement
A perfectly planned and well thought out data science project can’t hit the road until the stakeholders are not involved or fully satisfied. To achieve this harmonious goal, you must first arrange a meeting with the stakeholders and then hear out their perspectives. When you are all done with it then place before them your findings and analytics and whatnot and convince them that this laid out plan or strategy that you have been working on is the most tentative that should be practiced right now. Have enough evidence on you through which you can convince your stakeholders.
The data science training is the most ambient thing that you need to practice in order to become the best version of your professional self that can achieve higher ranks of this career.