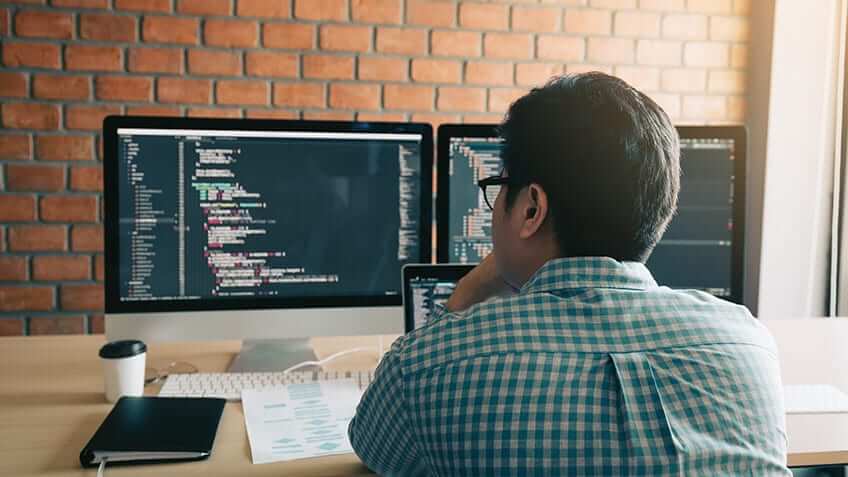
Reasons Why Banking System Needs More Data Scientists
Organizations need data to create bits of knowledge and settle on data-driven choices. To offer better types of assistance to its clients and devise techniques for different financial tasks, data science is a compulsory prerequisite. Moreover, banks need data to develop their business and draw more clients. We experience a portion of the significant zones where banking enterprises use data science to improve their products. We also see a significant part of data science in financial areas.
Data scientists have generally gone through 4+ years of education in computer science, statistics, math, or even physics and are regularly drawn closer by various scouts to work in a bank. A tremendous extent of graduates needs to work for a tech goliath like Google or Amazon and work on truly amazing projects. Nonetheless, our data science roles in finance limited since people are ignorant of the cutting-edge projects these organizations are doing that are hugely affecting their everyday life? Is it conceivable they don't know about the work being done in this domain? Enroll in our Data Science and Analyst bootcamp to get your data science career journey started.
Data has frequently been alluded to as the soul of the monetary area.
Picture this, you stroll into your nearby bank office. As you stroll in, reference points introduced sense a sign coming from your cell phone or you sign on to your internet banking, and your cell phone filters your finger impression or your eye and awards you access to your records without recalling passwords and the bank staff will have all the data about you effectively continuously. These knowledge frameworks are directed, refreshed, and created by a group of data scientists and the technology is frequently supported with AI strategies and big data handling.
At Capital One, for instance, data science calculations have been having a significant effect by building up the up-and-coming age of monetary products and administrations which cover almost 60 million clients. Capital One was an early adopter of analytics and has been utilizing data science to customize every Mastercard since 1988. This utilization of analytics has assisted with advancing the bank's development by over 20% profit per share making Capital One the third biggest supplier of MasterCard in the US. This would not have been conceivable without the interest in data scientists.
At Credit Suisse, data scientists are applying data mining methods and measurable analytics to construct top-notch hazard observing arrangements, which help distinguish new and arising hazard territories in the Bank. Data scientists are additionally being used across the business to discover novel occasions to make income streams, hold clients, and decrease costs.
There has been a gigantic pattern as of late inside banking where data scientists are assisting with dispatching and making new applications from data platforms. These applications can help monetary firms join data into each choice they make; they can computerize data digging and prescient demonstrating for everyday use and weave progressed measurable analysis, machine learning, and artificial intelligence into the bank's everyday tasks. This is additional verification of how significant the job of a data scientist is in the present finance environment.
A portion of the major banks is fabricating new interior tech bunches that are set up like a technology organization. The ultimate objective here is to give data scientists (and software engineers) a stage and working climate that is more natural to them and far eliminated from the prosaic culture everybody assumes is the situation in banking. The spotlight here is regularly on open-source technology and greenfield projects.
Data scientists are having an enormous impact in fighting payment fraud. Forestalled frauds aggregate in the billions of dollars consistently and this is regularly down to the prescient frameworks that data scientists have assembled and the calculations they have planned. Each bank on the planet has a group of data scientists that ensure their clients’ security.
In the advanced financial climate, buyers are very much educated. They expect instinctive, connective, and instructive encounters when they bank. Banks need solutions that can help them please their clients with customized encounters, enable their labor force to give separated encounters, streamline risk-taking abilities with AI-empowered experiences, and change services and products with data at the center. Applied data science and cloud-native business engineering are both basic for digital change for banks.
At the focal point of this change is the data researcher and the supporting group of data analysts and data engineers contingent upon the size of the association, and leading all these data personas is the main data official. The greatest test data researchers and groups face is conveying business results and taking experiences, models, and smart applications to make the business’ worth and show associations a solid business sway.
Extraordinary AI Needs Incredible Data
A genius data researcher will rush to understand the assumptions and will utilize incredible data to understand the estimation of AI. It's what we call "extraordinary AI needs incredible data." One must have an all-encompassing, coordinated perspective on the business and a mix of specialized abilities. The measuring stick for a star data researcher is a specialist who keeps up their prescribed procedures and is completely equipped for executing a data science cycle of self-assertive multifaceted nature from business ideation to arrangement. The genius data researcher has a solid device and language inclination, and they can discover or make arrangements utilizing accessible open-source or restrictive libraries. They are also happy to utilize a huge assortment of tools to address a particular need, regardless of whether they fall outside their inclination.
To be successful, the genius data researcher needs instruments that help them:
- Band together with business partners to see needs and distinguish occasions to apply data science by outlining the chance as a data science issue, detailing speculations and picking methods for experimentation
- View and comprehend business data in setting, while simultaneously dealing with data scale and multifaceted nature issues; additionally, recognizing and making business highlights for investigation, especially an objective variable
- Oversee and switch between figure conditions to scale out and scale up process with suitable calculation resources, for example, GPUs
- Simplify execution and generation of numerous varieties to issues by overseeing and understanding measurements through running different trials and making various models and different antiquities
- Convey arrangements into creation, clarifying outcomes alongside highlight and data heredity
Enroll in our Data Science and Analyst bootcamp to get your data science career journey started. Connect with our experts to learn more on how QuickStart can get you started with data science training.
Consolidate Data and AI on a Cloud-Native Architecture
We should move away from giving a bunch of different instruments and innovations to data analysts or data engineers with the expectation of upgrades toward their capacities. How about we move to an all-encompassing arrangement that empowers data researchers to embrace innovations as they become accessible, gathering gradual incentive to their cycles and work processes while adjusting them to business needs and present-day advancements.
Endeavor data can be siloed across many frameworks, such as data warehouses, databases, data pools, and file systems that are not AI-empowered. This implies a colossal measure of time is spent consolidating, cleaning, confirming, and advancing the data to prepare it for the model.
Artificial intelligence frameworks, such as PyTorch, TensorFlow, SciKit-Learn, don't do data training. They expect that datasets are spotless and have a pre-fabricated data framework to perform data handling. These technology silos make it difficult for endeavors to prevail in AI without a multitude of profoundly complex architects and data analysts and scientists. The capacity to utilize cloud-native architecture with the ability to scale here and there in the ecosystems of containers and microservices to send AI applications is central.
IBM Cloud Private for Data is the primary integrated analytics platform to bring data and AI advances together in a containerized platform. It could turn into the true data training and AI engine in projects today as a result of its speed, convenience, and modern analysis.
IBM is running an effective pilot and is probably the greatest bank in Asia. It offers recent college grads more noteworthy admittance to credit by creating inventive AI models that touch all parts of its loaning business. These models cycle a great many things of semi-organized data alongside organized data that applies a modern data science model to do forecasts.
The Requirement for Data Scientists in the Banking Sector
This position has the extraordinary capacity to utilize technology to decrease expenses and increment benefits, which makes them especially popular.
In deals, promoting, and publicizing, data science assists with focusing on clients better and decrease beat. These organizations are additionally going to data for risk checking and appraisal, financial modeling, trade surveillance, and fraud prevention. These territories rely upon representatives who can make, convey, and keep up calculations in the monetary space. One position that will in general cover with the monetary data scientist is the financial architect.
The Absence of Data Scientists in the Banking Sector
There are a lot of specialty positions in financial data science, and enormous banks are as of now scaling up groups of financial data scientists that can work solidly to add business esteem. Since it's amazingly uncommon to locate a senior financial data scientist, many are enrolling applicants with specific abilities that can help drive data-driven worth.
The skill gap in data science is clear. Colleges and universities have been delayed to bring to the table this course of study, which is the reason many decide online courses and data science bootcamps to learn data science. Given the surplus of money in data science courses, it's no big surprise this position is sought after.
Connect with our experts to learn more on how QuickStart can get you started with data science training.